-
-
Notifications
You must be signed in to change notification settings - Fork 15.9k
New issue
Have a question about this project? Sign up for a free GitHub account to open an issue and contact its maintainers and the community.
By clicking “Sign up for GitHub”, you agree to our terms of service and privacy statement. We’ll occasionally send you account related emails.
Already on GitHub? Sign in to your account
Interpreting training results and showing loss stats/graph #1468
Comments
Hello @naveedri, thank you for your interest in 🚀 YOLOv5! Please visit our ⭐️ Tutorials to get started, where you can find quickstart guides for simple tasks like Custom Data Training all the way to advanced concepts like Hyperparameter Evolution. If this is a 🐛 Bug Report, please provide screenshots and minimum viable code to reproduce your issue, otherwise we can not help you. If this is a custom training ❓ Question, please provide as much information as possible, including dataset images, training logs, screenshots, and a public link to online W&B logging if available. For business inquiries or professional support requests please visit https://www.ultralytics.com or email Glenn Jocher at glenn.jocher@ultralytics.com. RequirementsPython 3.8 or later with all requirements.txt dependencies installed, including $ pip install -r requirements.txt EnvironmentsYOLOv5 may be run in any of the following up-to-date verified environments (with all dependencies including CUDA/CUDNN, Python and PyTorch preinstalled):
StatusIf this badge is green, all YOLOv5 GitHub Actions Continuous Integration (CI) tests are currently passing. CI tests verify correct operation of YOLOv5 training (train.py), testing (test.py), inference (detect.py) and export (export.py) on MacOS, Windows, and Ubuntu every 24 hours and on every commit. |
GIoU term is deprecated: #1120 Follow custom training tutorial: |
I have already followed the custom training tutorial, but I still don't know what each graph in the results.png says. Is there a loss graph? How should I measure the results? |
@naveedri oh, for the metrics I would recommend the original YOLOv3 paper to brush up on the basics: and wikipedia for metrics: |
Thank you so much!
Here are the results I got on this (again, single image, 200 epochs |
You require a valid dataset for valid results. |
This issue has been automatically marked as stale because it has not had recent activity. It will be closed if no further activity occurs. Thank you for your contributions. |
I'm new to yolov5 and I'm trying to build a single class object detector(detecting mouth in an image).
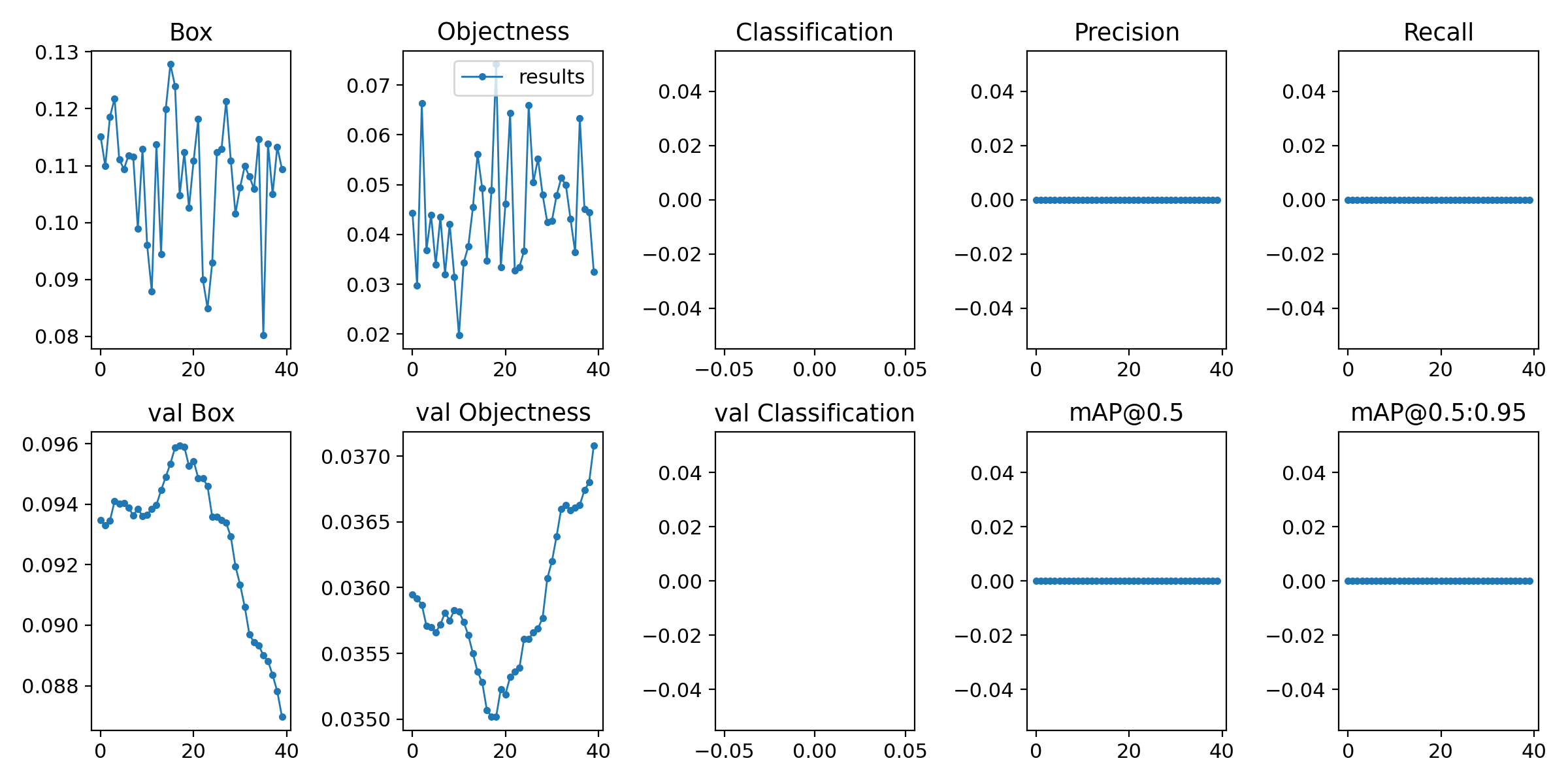
Here are the results I got:
I'm pretty sure that my dataset is problematic and thus the training wasn't successful, but putting this aside, I have two questions:
PS - I have also tried running train.py on a dataset that includes a single image of my mouth labeled - the same image is used for training, validation, and testing. I expected the model to be able to learn to label this image correctly as it is the only data it gets, but when it comes to testing or validation - the model isn't able to detect anything above 0.05 confident rate after 200 epochs - and even then it draws random boxes and doesn't detect the mouth correctly. Is it the expected result, or does it indicates that something is wrong in my setup?
The text was updated successfully, but these errors were encountered: