-
Notifications
You must be signed in to change notification settings - Fork 30
New issue
Have a question about this project? Sign up for a free GitHub account to open an issue and contact its maintainers and the community.
By clicking “Sign up for GitHub”, you agree to our terms of service and privacy statement. We’ll occasionally send you account related emails.
Already on GitHub? Sign in to your account
OneCycle LR, LR finder, custom Tensorboard, etc. #70
Conversation
This commit also includes - Custom tensorboard callback logging learning rate & momentum - A utils.py file collecting utilities used in more than one file - Clean-up of how output files are organized - Configuration files using the OneCycle scheduler
`mlpf/pipeline.py` is the beginning of a `click` based alternative to the `mlpf/launcher.py`.
Also add option to give a prefix to the name of the training directory
Also add lr_schedule parameter to configuration files
The previous commit still scaled the LR, this one fixes it.
- create get_train_val_datasets() function to get datasets for training - move targets_multi_output() from model_setup.py to utils.py for more flexible access (solving import loop issue)
The learning rate finder implements a technique to easily estimate a range of learning rates that should perform well given the current model setup. When the model architecture or other hyperparameters are changed, the learning rate finder can be run in order to find a new suitable LR range. The learning rate finder starts training the model at a very low LR, increasing it every batch. The batch loss is plotted vs training steps and a figure is created from which a suitable LR range can be determined. This technique was first introduced by Leslie Smith in https://arxiv.org/abs/1506.01186.
I think the new pipeline and the reorganization are great! |
Thanks! |
When running `python mlpf/pipeline.py evaluate -t <train_dir>` without specifying which weights to use explicitly the pipeline will load the weights with the smallest loss in <train_dir>/weights/ that it can find.
This can be useful when many large checkpoint files take up too much storage space.
The default parameters for expdecay added to the config files in this commit are the same as those used on the jpata/particleflow master branch at the time of writing.
Also: - Add missing parameters to config files. - Move make_weights_function to utils.py
There was a problem hiding this comment.
Choose a reason for hiding this comment
The reason will be displayed to describe this comment to others. Learn more.
Looks good, a small comment inline.
I think we could go ahead with this, and later follow up with a PR that completely moves all functionality to the new pipeline (I didn't try the new one yet, just made sure the old pipeline works as before).
Thanks a lot for the effort!
OneCycle LR, LR finder, custom Tensorboard, etc. Former-commit-id: 1e4c581
This pull request includes several new features.
OneCycleScheduler
The OneCycleScheduler is a
tf.keras.optimizers.schedules.LearningRateSchedule
that schedules the learning rate on a 1cycle policy as per Leslie Smith's paper (https://arxiv.org/pdf/1803.09820.pdf). The implementation adopts additional improvements as per the fastai library (https://docs.fast.ai/callbacks.one_cycle.html) where only two phases are used and the adaptation is done using cosine annealing.In my experience the OneCycle policy improves generalization and speeds up learning.
Learning Rate Finder
The learning rate finder implements a technique to easily estimate a range of learning rates that should perform well given the current model setup. When the model architecture or other hyperparameters are changed, the learning rate finder can be run in order to find a new suitable LR range.
The learning rate finder starts training the model at a very low LR, increasing it every batch. The batch loss is plotted vs LR (or, equivalently, training steps) and a figure is created from which a suitable LR range can be determined.
This technique was first introduced by Leslie Smith in
https://arxiv.org/abs/1506.01186.
Simply run
and a plot of loss vs learning rate like the one below will be created. A suitable LR range lies somewhere in the negative slope of the curve, where the loss is steadily decreasing at a relatively high rate.
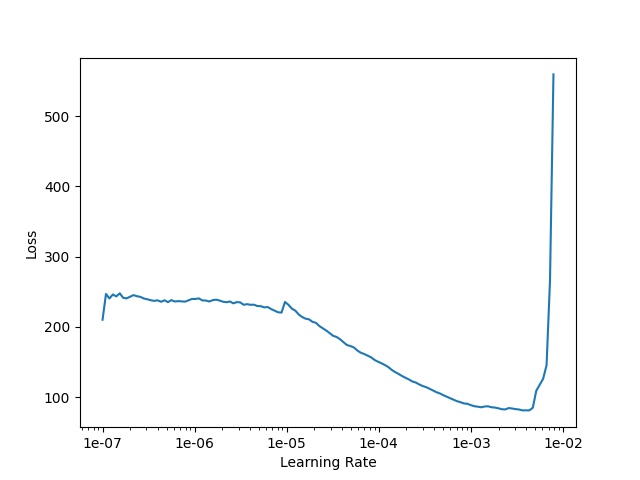
Pipeline.py
mlpf/pipeline.py
is my beginning of aclick
based alternative to themlpf/launcher.py
. I created it, in part, to not change themlpf/launcher.py
too much in one pull request. Ifmlpf/pipeline.py
is well liked it might be able to replacemlpf/launcher.py
sometime in the future. Right now, it is still a work in progress.Other notes
The learning rate is no longer scaled by the batch size. Instead, the learning rate used is now the same as explicitly defined in the configuration file. When using the exponential decay schedule or the OneCycle schedule, the LR specified in the config will be the maximum LR used in the schedule.
The structure of the training directory has been reorganized. Instead of writing many files directly in the training directory they have been organized in different subfolders:
history
: contains the history_{}.json, event_{}.pdf and cm_normed.pdf filesweights
: contains all checkpoints of model weightsevaluation
: contains the pred.npz file(s)tensorboard_logs
: contains the tensorboard logs