-
-
Notifications
You must be signed in to change notification settings - Fork 15.9k
New issue
Have a question about this project? Sign up for a free GitHub account to open an issue and contact its maintainers and the community.
By clicking “Sign up for GitHub”, you agree to our terms of service and privacy statement. We’ll occasionally send you account related emails.
Already on GitHub? Sign in to your account
Calculation of Centroids from the Detection Results #6636
Comments
👋 Hello @TehseenHasan, thank you for your interest in YOLOv5 🚀! Please visit our ⭐️ Tutorials to get started, where you can find quickstart guides for simple tasks like Custom Data Training all the way to advanced concepts like Hyperparameter Evolution. If this is a 🐛 Bug Report, please provide screenshots and minimum viable code to reproduce your issue, otherwise we can not help you. If this is a custom training ❓ Question, please provide as much information as possible, including dataset images, training logs, screenshots, and a public link to online W&B logging if available. For business inquiries or professional support requests please visit https://ultralytics.com or email support@ultralytics.com. RequirementsPython>=3.7.0 with all requirements.txt installed including PyTorch>=1.7. To get started: git clone https://github.com/ultralytics/yolov5 # clone
cd yolov5
pip install -r requirements.txt # install EnvironmentsYOLOv5 may be run in any of the following up-to-date verified environments (with all dependencies including CUDA/CUDNN, Python and PyTorch preinstalled):
StatusIf this badge is green, all YOLOv5 GitHub Actions Continuous Integration (CI) tests are currently passing. CI tests verify correct operation of YOLOv5 training (train.py), validation (val.py), inference (detect.py) and export (export.py) on MacOS, Windows, and Ubuntu every 24 hours and on every commit. |
@TehseenHasan nice work! |
👋 Hello, this issue has been automatically marked as stale because it has not had recent activity. Please note it will be closed if no further activity occurs. Access additional YOLOv5 🚀 resources:
Access additional Ultralytics ⚡ resources:
Feel free to inform us of any other issues you discover or feature requests that come to mind in the future. Pull Requests (PRs) are also always welcomed! Thank you for your contributions to YOLOv5 🚀 and Vision AI ⭐! |
hello @glenn-jocher Load YOLOv8 modelmodel = YOLO("C:\Users\manar\OneDrive\Desktop\roboflow\runs\detect\train\weights\best.pt") Check if model loaded successfullyif model is None: Configuring Modelmodel.conf = 0.25 # NMS confidence threshold Function to draw Centroids on the detected objects and return updated imagedef draw_centroids_on_image(output_image, detections):
if name == "main":
|
i want with this code to give me the possition of the object detected i worked yolov8 . |
Hi there! If you're using YOLOv8 and want to extract the position of the detected objects, your approach seems almost correct. However, it appears there might be a misunderstanding in accessing the output. Here's a corrected snippet on how you might process the detection results to extract positions: results = model(image) # Perform inference
detections = results.xyxy[0] # Extract detections; xyxy returns [xmin, ymin, xmax, ymax, confidence, class]
for detection in detections:
xmin, ymin, xmax, ymax, conf, cls = detection
cx = (xmin + xmax) / 2
cy = (ymin + ymax) / 2
# Now you can use cx, cy as the centroid coordinates Ensure that you convert the tensor to numpy if necessary and handle the data types appropriately. This should give you the centroid positions of the bounding boxes. If you encounter any further issues, feel free to ask! Happy coding! 😊 |
hello @glenn-jocher , i am work in arm robot (pick and place ) with 4 DOF how can i use this value to change it to an order to pick the object detected ( i work it with yolov8) |
Hello! To integrate YOLOv8 detections with your 4-DOF robot for a pick-and-place task, you'll need to translate the detected object's centroid coordinates into robot arm commands. Here's a simplified approach:
Sample code snippet: results = model(image)
detections = results.xyxy[0] # Detections with [xmin, ymin, xmax, ymax, confidence, class]
for detection in detections:
xmin, ymin, xmax, ymax, conf, cls = detection
# Calculate centroid
cx = (xmin + xmax) / 2
cy = (ymin + ymax) / 2
# Convert to real-world coordinates (assuming simple scale factor for demo)
real_x = scale_factor * cx
real_y = scale_factor * cy
# Generate robot arm commands based on converted coordinates
robot_arm.move_to(real_x, real_y) Make sure to replace Feel free to reach out if you need more detailed help or specific adjustments. Happy building! 😊 |
hello @glenn-jocher, u good ?I used this code to measure the angle to my detected object, but it seems to be producing inaccurate values. What can I do to correct it? Set custom confidence threshold for the 'person' classconfidence_threshold = 0.1 Load yolov8 modelmodel = YOLO("C:\Users\manar\OneDrive\Desktop\roboflow\runs\detect\train\weights\best.pt") Load webcamcap = cv2.VideoCapture(0) # 0 for the default webcam; adjust the index if you have multiple webcams Camera-specific parameters (replace with your actual values)horizontal_fov_degrees = 90 Main processing loopwhile True:
Release resourcescap.release() |
Hello! If you're experiencing inaccuracies in angle measurement from your detected object, here are a few things you might consider checking or adjusting:
Here’s a tiny refactor to ensure FOV adjustments based on resized frame dimensions: x_degrees = (centroid_x / 960) * horizontal_fov_degrees # Use resized width
y_degrees = (centroid_y / 540) * vertical_fov_degrees # Use resized height Make these adjustments and see if your angle measurements improve! 😊 If you’re still facing issues, ensure the centroid calculation alignment with the detection bounding box is accurate and consistent. |
Hello, I see there are some people searching and asking here how to calculate the centroids from the detected objects. I have written some demo code for that purpose. If anyone has this requirement you can check my code. Thanks!
Link: https://github.com/TehseenHasan/yolo5-object-detection-and-centroid-finding
The output of my code is:
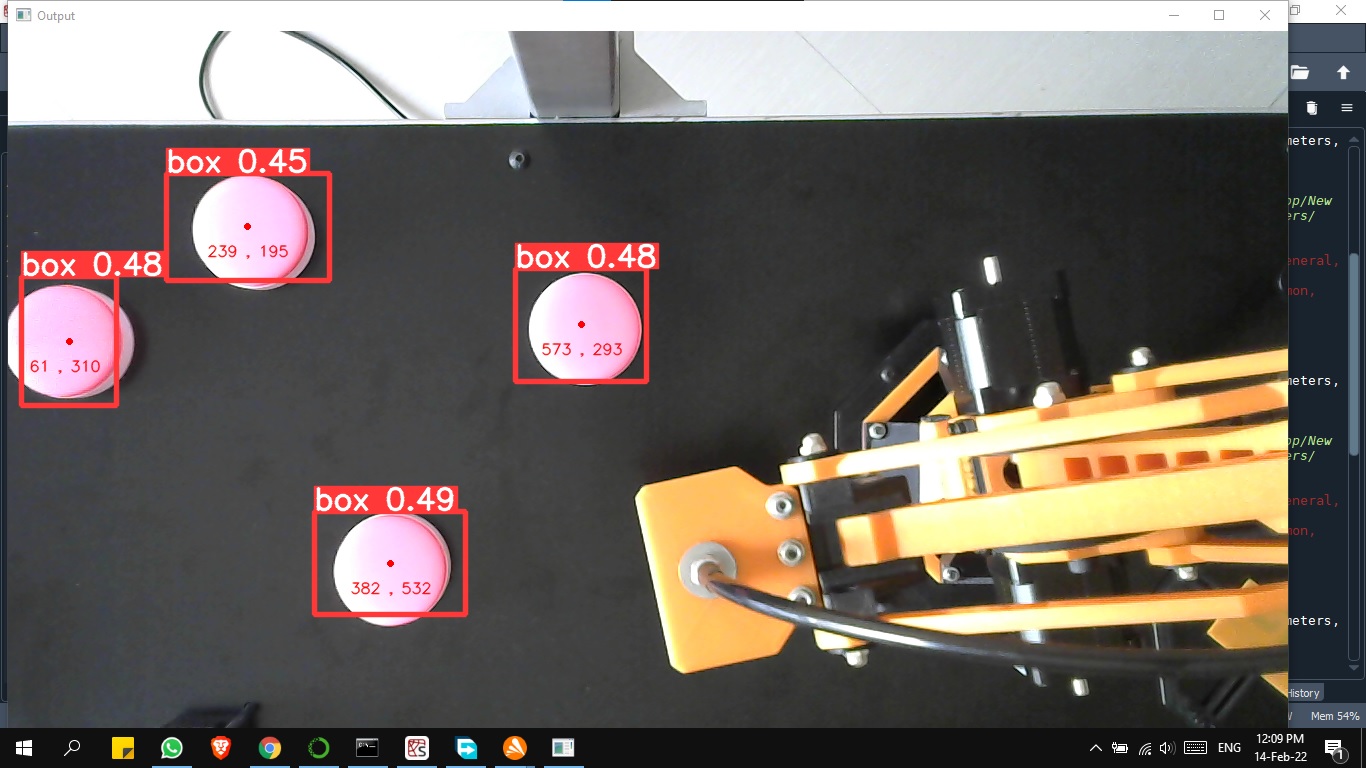
The text was updated successfully, but these errors were encountered: