We read every piece of feedback, and take your input very seriously.
To see all available qualifiers, see our documentation.
Have a question about this project? Sign up for a free GitHub account to open an issue and contact its maintainers and the community.
By clicking “Sign up for GitHub”, you agree to our terms of service and privacy statement. We’ll occasionally send you account related emails.
Already on GitHub? Sign in to your account
I noticed that the output gradient is pretty small (1e-14~1e-7) while much bigger (1e-8~1e-1) in the https://github.com/PAIR-code/saliency implementation.
First, I tried this notebook: https://github.com/PAIR-code/saliency/blob/master/Examples.ipynb and output the min/max of vanilla gradient and the top 20.
np.max(vanilla_mask_3d), np.min(vanilla_mask_3d)
(0.3862362, -0.38338542)
np.sort(vanilla_mask_3d.ravel())[::-1][:20]
array([0.3862362 , 0.36115727, 0.33639386, 0.3209837 , 0.32015106, 0.31286812, 0.3043984 , 0.3020323 , 0.29084682, 0.28999907, 0.28522477, 0.27706975, 0.27350807, 0.27175996, 0.2703794 , 0.2685724 , 0.26725614, 0.26650152, 0.26406616, 0.26123673], dtype=float32)
But when I run it using deep-viz-keras with the same model and same image, the result looks like this:
np.max(mask), np.min(mask)
(2.2573392039149098e-07, -4.179406574422728e-07)
np.sort(mask.ravel())[::-1][:20]
array([2.25733920e-07, 2.23271692e-07, 1.98080411e-07, 1.92421204e-07, 1.90237181e-07, 1.86172134e-07, 1.81749829e-07, 1.76881710e-07, 1.75296812e-07, 1.75214721e-07, 1.74797302e-07, 1.73955064e-07, 1.71986304e-07, 1.71514253e-07, 1.67088409e-07, 1.66170971e-07, 1.66098115e-07, 1.65983923e-07, 1.65190807e-07, 1.61611446e-07]).
Also, the output mask is not exactly the same.
Here's the PAIR/saliency version:
PAIR/saliency
Here's the deep-vis-keras version:
deep-vis-keras
Here's the notebook code to set deep-viz-keras in the same setting as PAIR/saliency. Notice I used the doberman image from the PAIR/saliency repo (https://github.com/PAIR-code/saliency/blob/master/doberman.png).
slim=tf.contrib.slim if not os.path.exists('models/research/slim'): !git clone https://github.com/tensorflow/models/ old_cwd = os.getcwd() os.chdir('models/research/slim') from nets import inception_v3 os.chdir(old_cwd) if not os.path.exists('inception_v3.ckpt'): #!wget http://download.tensorflow.org/models/inception_v3_2016_08_28.tar.gz !curl -O http://download.tensorflow.org/models/inception_v3_2016_08_28.tar.gz !tar -xvzf inception_v3_2016_08_28.tar.gz ckpt_file = './inception_v3.ckpt' graph = tf.Graph() with graph.as_default(): images = tf.placeholder(tf.float32, shape=(None, 299, 299, 3)) with slim.arg_scope(inception_v3.inception_v3_arg_scope()): _, end_points = inception_v3.inception_v3(images, is_training=False, num_classes=1001) # Restore the checkpoint sess = tf.Session(graph=graph) saver = tf.train.Saver() saver.restore(sess, ckpt_file) # Construct the scalar neuron tensor. logits = graph.get_tensor_by_name('InceptionV3/Logits/SpatialSqueeze:0') neuron_selector = tf.placeholder(tf.int32) y = logits[0][neuron_selector] # Construct tensor for predictions. prediction = tf.argmax(logits, 1) from matplotlib import pylab as P # Boilerplate methods. def ShowImage(im, title='', ax=None): if ax is None: P.figure() P.axis('off') im = ((im + 1) * 127.5).astype(np.uint8) P.imshow(im) P.title(title) def LoadImage(file_path): im = PIL.Image.open(file_path) im = np.asarray(im) return im / 127.5 - 1.0 im = LoadImage('fig/doberman.png') # Show the image ShowImage(im) # Make a prediction. prediction_class = sess.run(prediction, feed_dict = {images: [im]})[0] print("Prediction class: " + str(prediction_class)) # Should be a doberman, class idx = 237 from keras.applications import inception_v3 model = inception_v3.InceptionV3(include_top=True, weights='imagenet') from saliency import GradientSaliency # TODO: how to create an Inception_v3 model here? vanilla = GradientSaliency(model) mask = vanilla.get_mask(im) show_image(mask, ax=plt.subplot('121'), title='vanilla gradient') mask = vanilla.get_smoothed_mask(im) show_image(mask, ax=plt.subplot('122'), title='smoothed vanilla gradient') print(np.max(mask), np.min(mask)) print(np.sort(mask.ravel())[::-1][:20])
Is this a bug? I'll appreciate if anyone can take a look.
The text was updated successfully, but these errors were encountered:
No branches or pull requests
I noticed that the output gradient is pretty small (1e-14~1e-7) while much bigger (1e-8~1e-1) in the https://github.com/PAIR-code/saliency implementation.
First, I tried this notebook: https://github.com/PAIR-code/saliency/blob/master/Examples.ipynb and output the min/max of vanilla gradient and the top 20.
np.max(vanilla_mask_3d), np.min(vanilla_mask_3d)
np.sort(vanilla_mask_3d.ravel())[::-1][:20]
But when I run it using deep-viz-keras with the same model and same image, the result looks like this:
np.max(mask), np.min(mask)
np.sort(mask.ravel())[::-1][:20]
Also, the output mask is not exactly the same.
Here's the
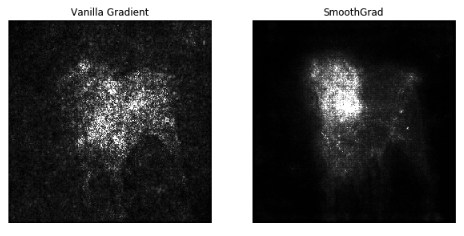
PAIR/saliency
version:Here's the
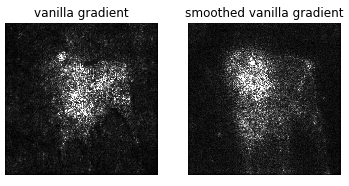
deep-vis-keras
version:Here's the notebook code to set deep-viz-keras in the same setting as PAIR/saliency. Notice I used the doberman image from the PAIR/saliency repo (https://github.com/PAIR-code/saliency/blob/master/doberman.png).
Is this a bug? I'll appreciate if anyone can take a look.
The text was updated successfully, but these errors were encountered: